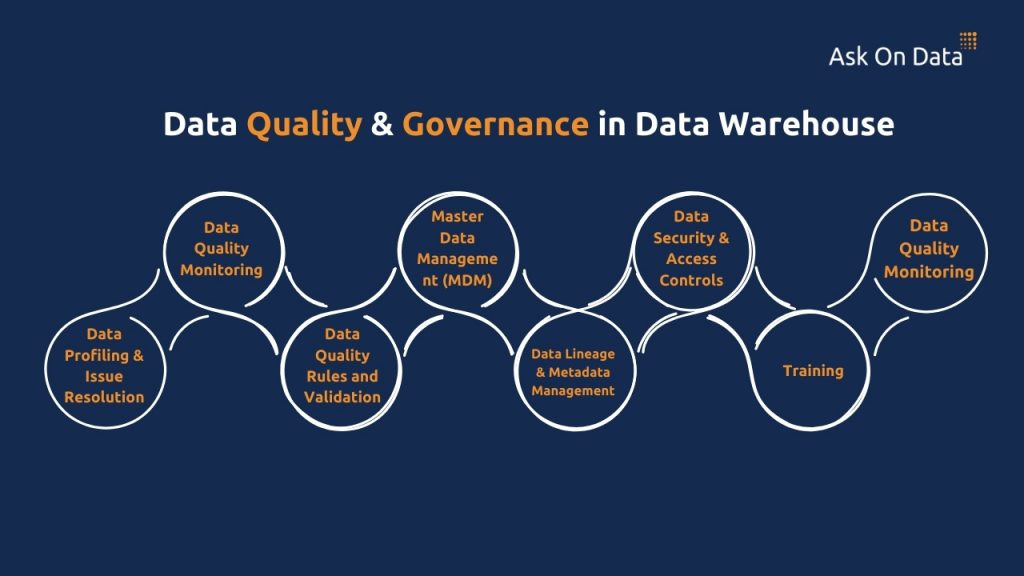
In this below blog we have highlighted the data quality and governance which should be followed when creating a data warehouse to have an enterprise ready data warehouse.
- Having a Data Governance Framework defining roles, responsibilities and processes for managing the DW and other assets related to the DW.
(B) Data Quality Rules and Validation:
– While creating the ETL jobs, have data quality rules and validation checks at various stages.
– Using data profiling tools to analyze and monitor data quality metrics, such as completeness, accuracy, consistency and uniqueness.
– Establish processes within ETL jobs for handling data quality issues, such as rejection, null data etc.
(C)Master Data Management (MDM):
– Implement a master data management (MDM) solution to create and maintain a single source of truth for critical master data entities, such as customers, products, locations etc.
(D) Data Lineage & Metadata Management:
– Establish and maintain a robust metadata management system that captures and documents data lineage, including the origin, transformations, and movement of data within the data warehouse.
– Data lineage and metadata facilitate impact analysis, auditing, and traceability, enabling better understanding and governance of data assets.
(E) Data Quality Monitoring:
– Implement data quality monitoring processes to continuously assess the quality of data within the data warehouse.
(F) Data Profiling & Issue Resolution:
– Conduct regular data profiling exercises to identify data quality issues, such as missing values, duplicates, or inconsistencies.
– Establish processes for investigating and resolving data quality issues, including root cause analysis and corrective actions.
(G) Data Security & Access Controls:
– Implement appropriate data security measures, such as access controls, encryption and auditing, to protect the integrity and confidentiality of data within the data warehouse.
– Ensure that access to data is granted only to authorized users and processes based on predefined roles and permissions (RBAC).
(H) Training:
– Provide training to IT team and making them aware of the best practices.
Tools like Ask On Data, with its simple chat interface powered by AI, can help you simply type and load the data into the data warehouse as well as do the required transformations. It can help in saving around 93% time in creating data pipelines as compared to tradition ETL tools.
If you are looking for some professional guidance you can reach out on www.helicaltech.com